Science rests on data, of that there can be no doubt. But peer through the hot haze of hype surrounding the use of big data in biology and you will see plenty of cold facts that suggest we need fresh thinking if we are to turn the swelling ocean of “omes” – genomes, proteomes and transcriptomes – into new drugs and treatments.
The relatively meager returns from the human genome project reflect how DNA sequences do not translate readily into understanding of disease, let alone treatments. The rebranding of “personalized medicine” – the idea that decoding the genome will lead to treatments tailored to the individual – as “precision medicine” reflects the dawning realisation that using the -omes of groups of people to develop targeted treatments is quite different from using a person’s own genome.
Because we are all ultimately different, the only way to use our genetic information to predict how an individual will react to a drug is if we have a profound understanding of how the body works, so we can model the way that each person will absorb and interact with the drug molecule. This is tough to do right now, so the next best thing is precision medicine, where we look at how genetically similar people react and then assume that a given person will respond in a similar way.
Even the long-held dream that drugs can be routinely designed by knowing the atomic structure of proteins, in order to identify the location in a protein where a drug acts, has not been realized.
Most importantly, the fact that “most published research findings are false,” as famously reported by John Ioannidis, an epidemiologist from Stanford University, underlines that data is not the same as facts; one critical dataset – the conclusions of peer reviewed studies – is not to be relied on without evidence of good experimental design and rigorous statistical analysis. Yet many now claim that we live in the “data age.” If you count research findings themselves as an important class of data, it is very worrying to find that they are more likely to be false (incorrect) than true.
“There’s no doubt of the impact of big data, which could contribute more than £200 billion to the UK economy alone over five years,” says Roger Highfield, director of external affairs at the Science Museum, London. But “the worship of big data has encouraged some to make the extraordinary claim that this marks the end of theory and the scientific method.”
Useful but not profound
The worship of big data downplays many issues, some profound. To make sense of all this data, researchers are using a type of artificial intelligence known as neural networks. But no matter their “depth” and sophistication, they merely fit curves to existing data. They can fail in circumstances beyond the range of the data used to train them. All they can, in effect, say is that “based on the people we have seen and treated before, we expect the patient in front of us now to do this.”
Still, they can be useful. Two decades ago, one of us (Peter) used big data and neural networks to predict the thickening times of complex slurries (semi-liquid mixtures) from infrared spectrums of cement powders. But, even though this became a commercial offering, it has not brought us one iota closer to understanding what mechanisms are at play, which is what is needed to design new kinds of cement.
The most profound challenge arises because, in biology, big data is actually tiny relative to the complexity of a cell, organ or body. One needs to know which data is important for a particular objective. Physicists understand this only too well. The discovery of the Higgs boson at CERN’s Large Hadron Collider required petabytes of data; nevertheless, they used theory to guide their search. Nor do we predict tomorrow’s weather by averaging historic records of that day’s weather – mathematical models do a much better job with the help of daily data from satellites.
Some even dream of minting new physical laws by mining data. But the results to date are limited and unconvincing. As Edward put it: “Does anyone really believe that data mining could produce the general theory of relativity?"
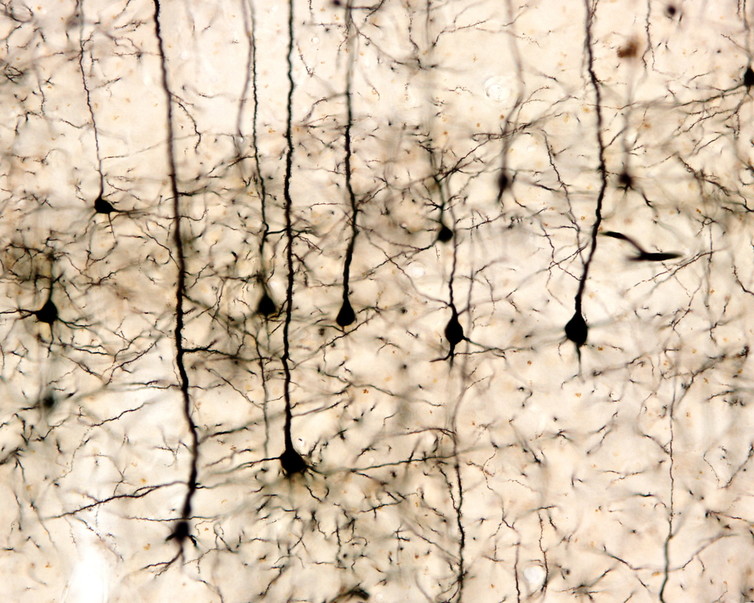
Understand laws of biology
Many advocates of big data in biology cling to the forlorn hope that we won’t need theory to form our understanding of the basis of health and disease. But trying to forecast a patient’s reaction to a drug based on the mean response of a thousand others is like trying to forecast the weather on a given date by averaging historic records of that day’s weather.
Equally, trying to find new drugs through machine learning based on accessing all known drugs and existing molecular targets is liable to fail because it is based on existing chemical structures and tiny changes in a potential drug can lead to dramatic differences in potency.
We need deeper conceptualization, but the prevailing view is that the complexities of life do not easily yield to theoretical models. Leading biological and medical journals publish vanishingly little theory-led, let alone purely theoretical, work. Most data provides snapshots of health, whereas the human body is in constant flux. And very few students are trained to model it.
To effectively use the explosion in big data, we need to improve the modelling of biological processes. As one example of the potential, Peter is already reporting results that show how it will soon be possible to take a person’s genetic makeup and – with the help of sophisticated modelling, heavyweight computing and clever statistics – select the right customised drug in a matter of hours. In the longer term, we are also working on virtual humans, so treatments can be initially tested on a person’s digital doppelganger.
But, to realize this dream, we need to divert funding used to gather and process data towards efforts to discern the laws of biology. Yes, big data is important. But we need big theory too.
By Peter Coveney, Professor of Physical Chemistry & Director of Centre for Computational Science, UCL and Edward R Dougherty, Distinguished professor, Texas A&M University. This article was originally published on The Conversation. Read the original article.